Introduction
The field of artificial intelligence has witnessed remarkable progress in Vision-Language Models (VLMs), which bridge the gap between visual and textual data. DeepSeek-VL2, the latest innovation in the DeepSeek series, sets a new benchmark in multimodal understanding by leveraging a Mixture-of-Experts (MoE) architecture. This model not only enhances performance but also optimizes efficiency, making it a standout in the competitive landscape of VLMs.
DeepSeek-VL2 introduces groundbreaking features such as a dynamic tiling vision encoding strategy and Multi-head Latent Attention (MLA), which enable it to process high-resolution images and complex textual data seamlessly. In this article, we delve into the architecture, data construction, training methodology, and evaluation of DeepSeek-VL2, showcasing its state-of-the-art capabilities.
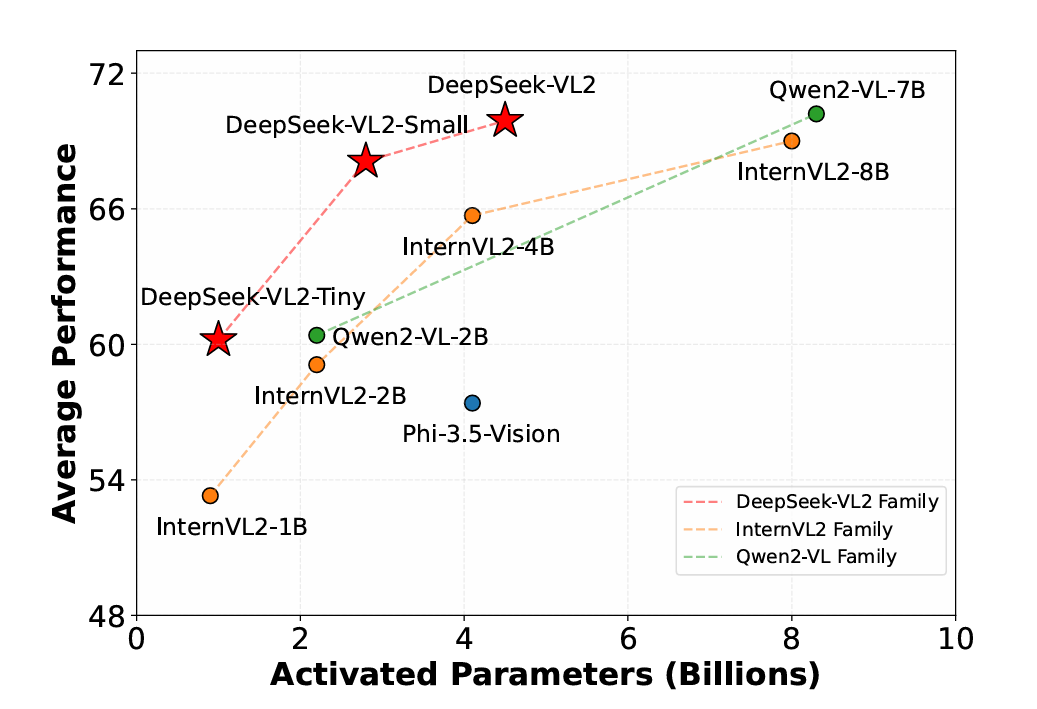
Model Architecture
At the heart of DeepSeek-VL2 lies a robust architecture comprising three core modules:
- Vision Encoder: Utilizes a dynamic tiling strategy to process images with varying aspect ratios efficiently.
- Vision-Language Adaptor: Acts as a bridge, aligning visual and textual embeddings for seamless integration.
- Mixture-of-Experts Language Model: Features Multi-head Latent Attention (MLA) for efficient and scalable inference.
The dynamic tiling strategy is a key innovation, allowing the model to handle high-resolution images without compromising on computational efficiency. This improvement over its predecessor, DeepSeek-VL, ensures superior performance across diverse visual inputs.
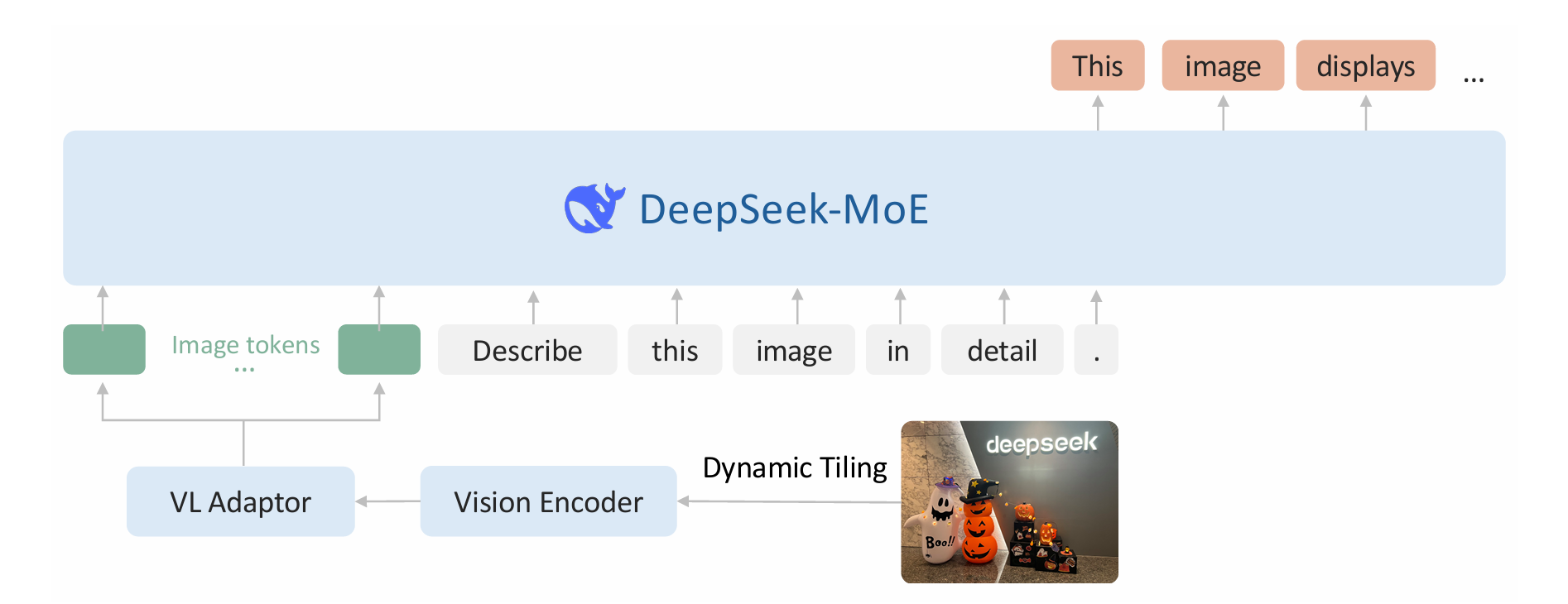

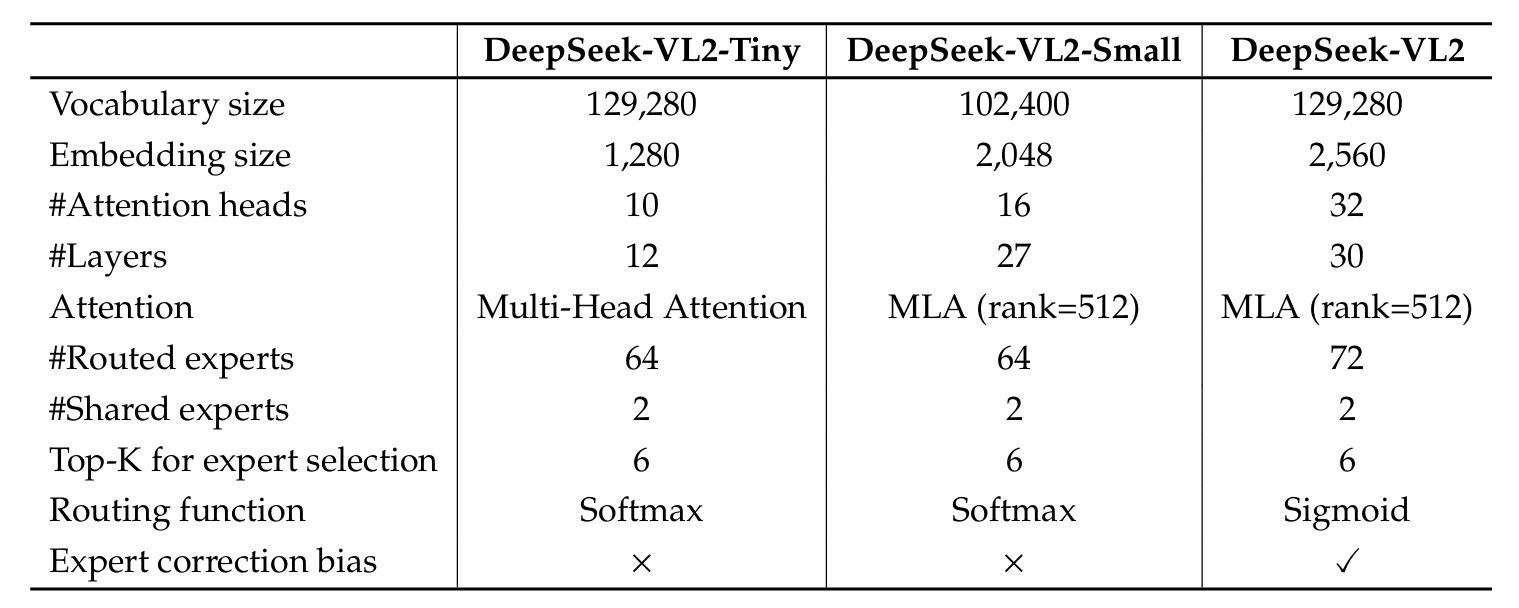
Data Construction
The training data for DeepSeek-VL2 is meticulously curated to enhance its multimodal capabilities. The data construction process is divided into three stages:
- Vision-Language Alignment Data: Focuses on aligning visual and textual embeddings for better integration.
- Vision-Language Pretraining Data: Combines vision-language datasets with text-only datasets to improve generalization.
- Supervised Fine-Tuning Data: Refines the model's instruction-following and conversational abilities.
The dataset sources include image captioning, optical character recognition (OCR), visual question answering (VQA), and visual grounding data, ensuring a diverse and comprehensive training foundation.
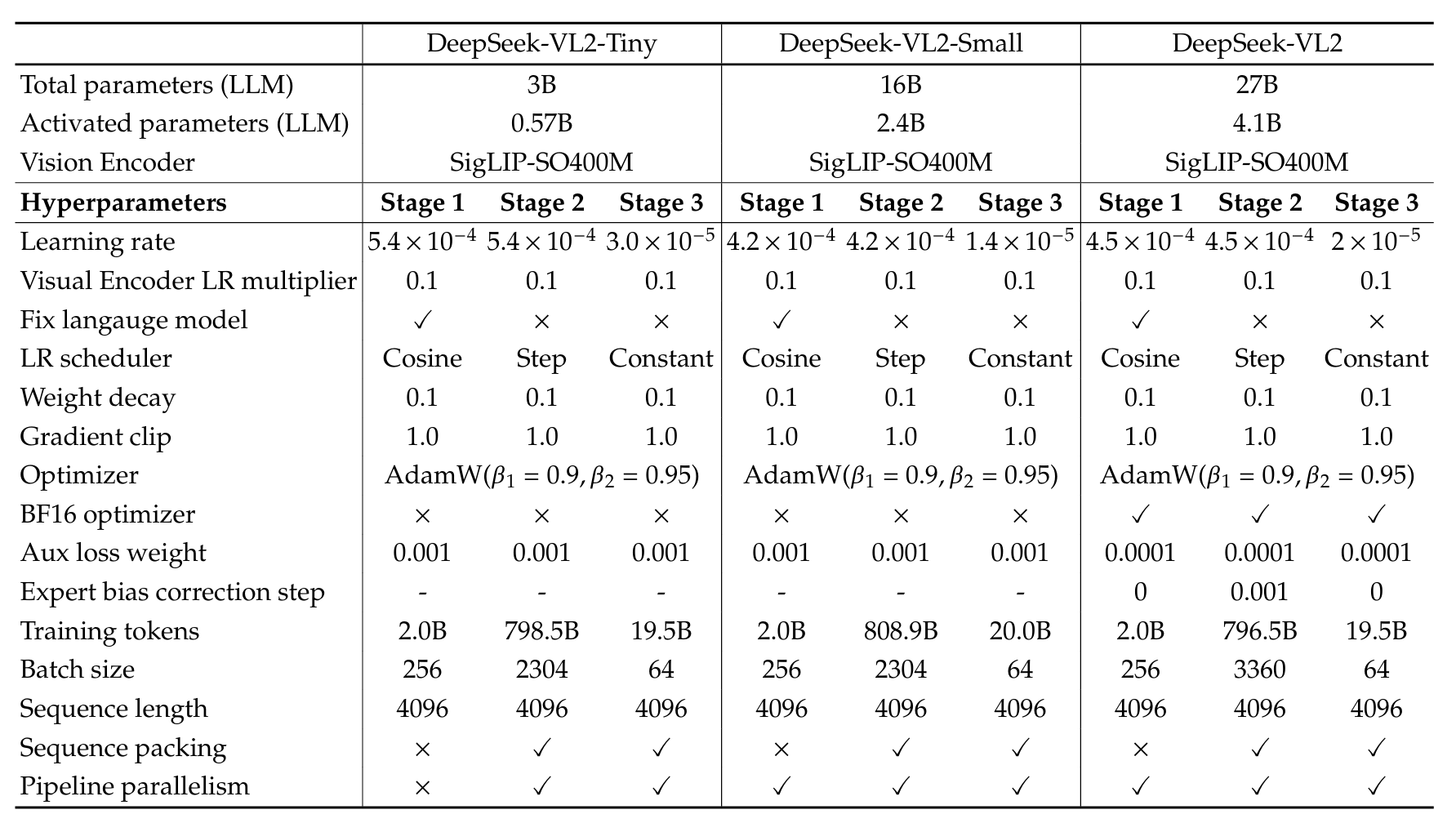
Training Methodology
DeepSeek-VL2 employs a three-stage training pipeline designed for efficiency and scalability:
- Vision-Language Alignment: Optimizes the vision encoder and adaptor while keeping the language model frozen.
- Vision-Language Pretraining: Unlocks all parameters for joint optimization, enhancing multimodal understanding.
- Supervised Fine-Tuning: Refines the model's ability to follow instructions and engage in grounded conversations.
The training process leverages advanced techniques such as pipeline parallelism and expert parallelism, ensuring efficient utilization of computational resources.
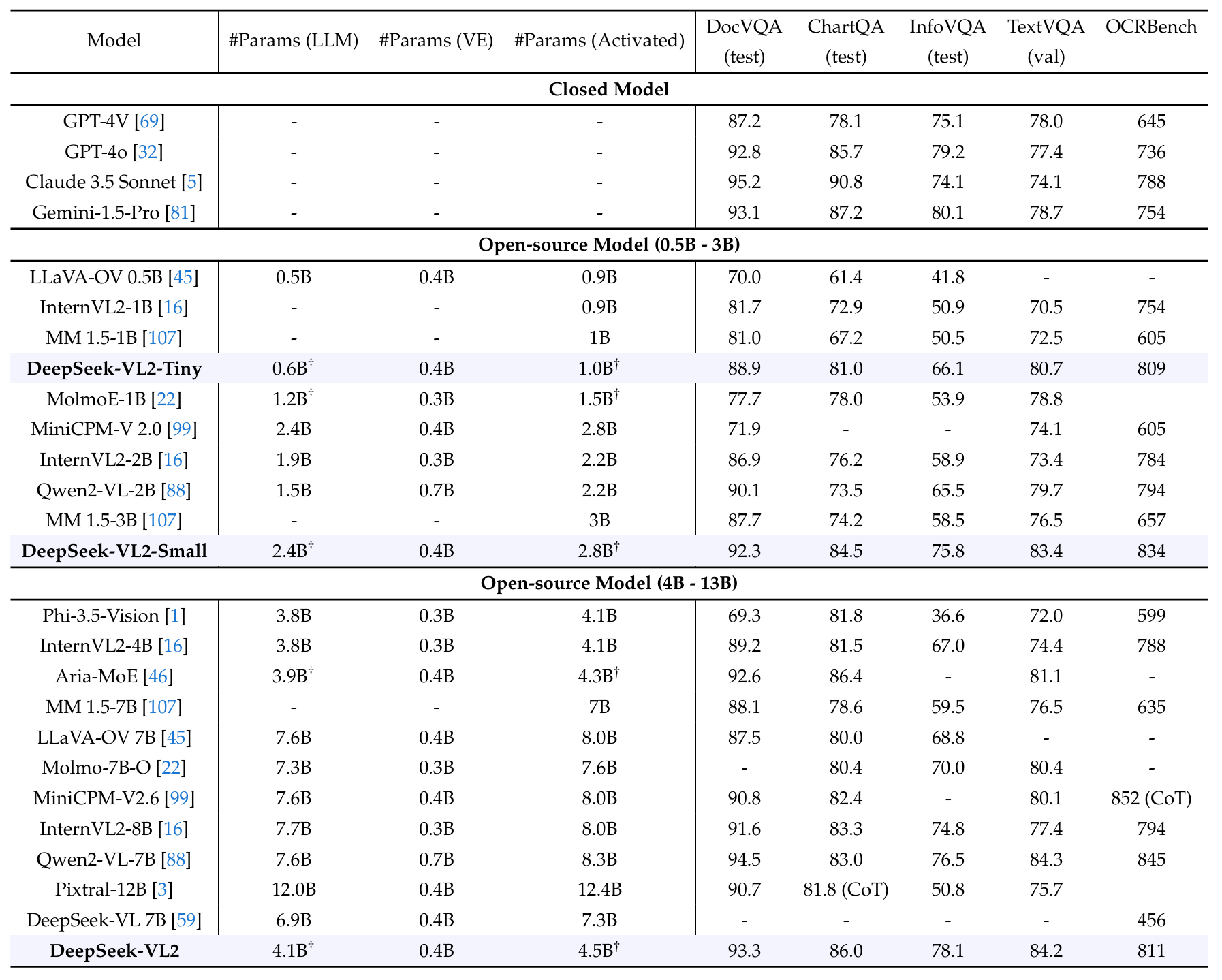
Evaluation
DeepSeek-VL2 sets a new standard in multimodal understanding, excelling across various benchmarks such as document understanding, chart interpretation, and visual reasoning. Its capabilities extend to creative tasks like visual storytelling, meme understanding, and grounded conversations.
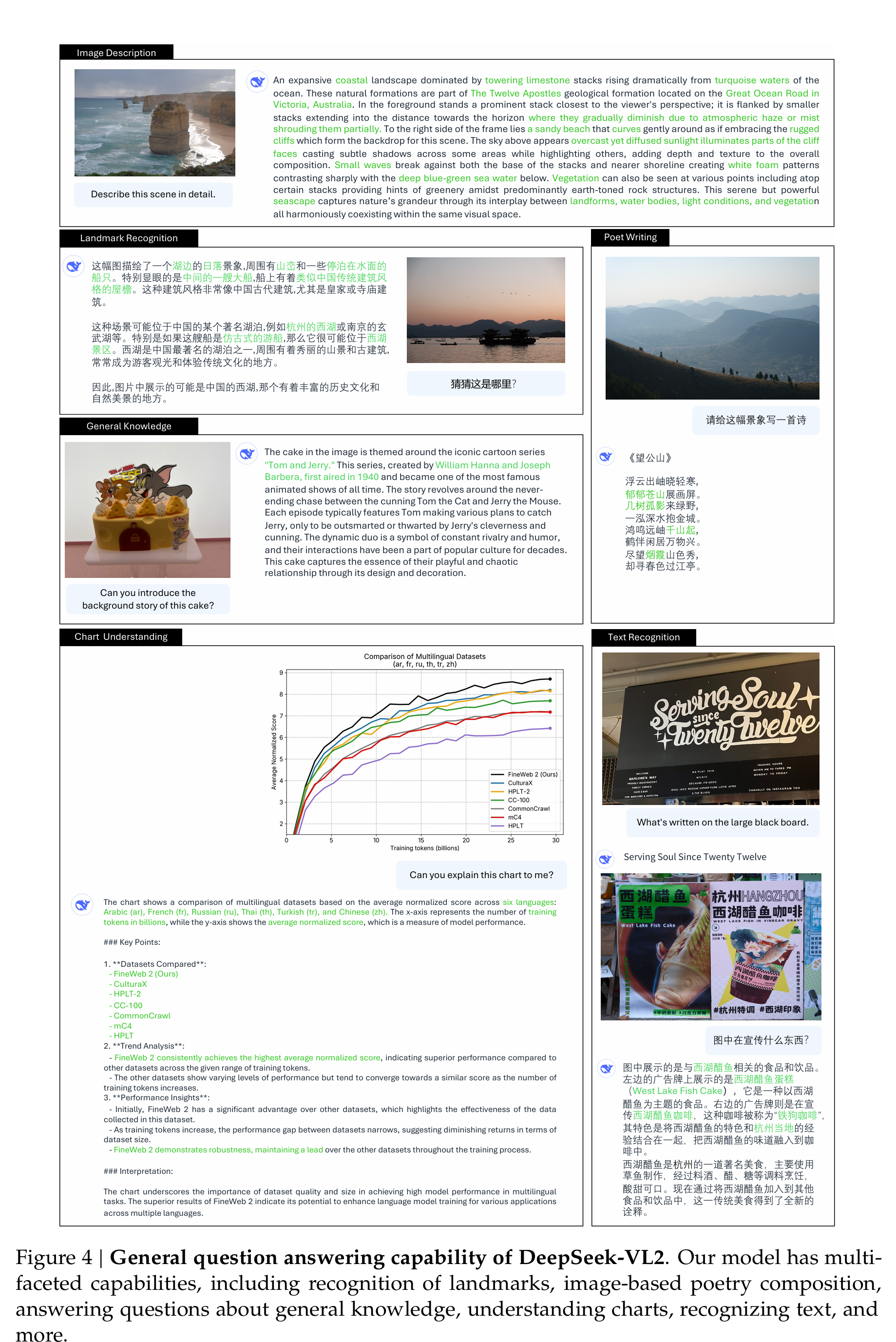
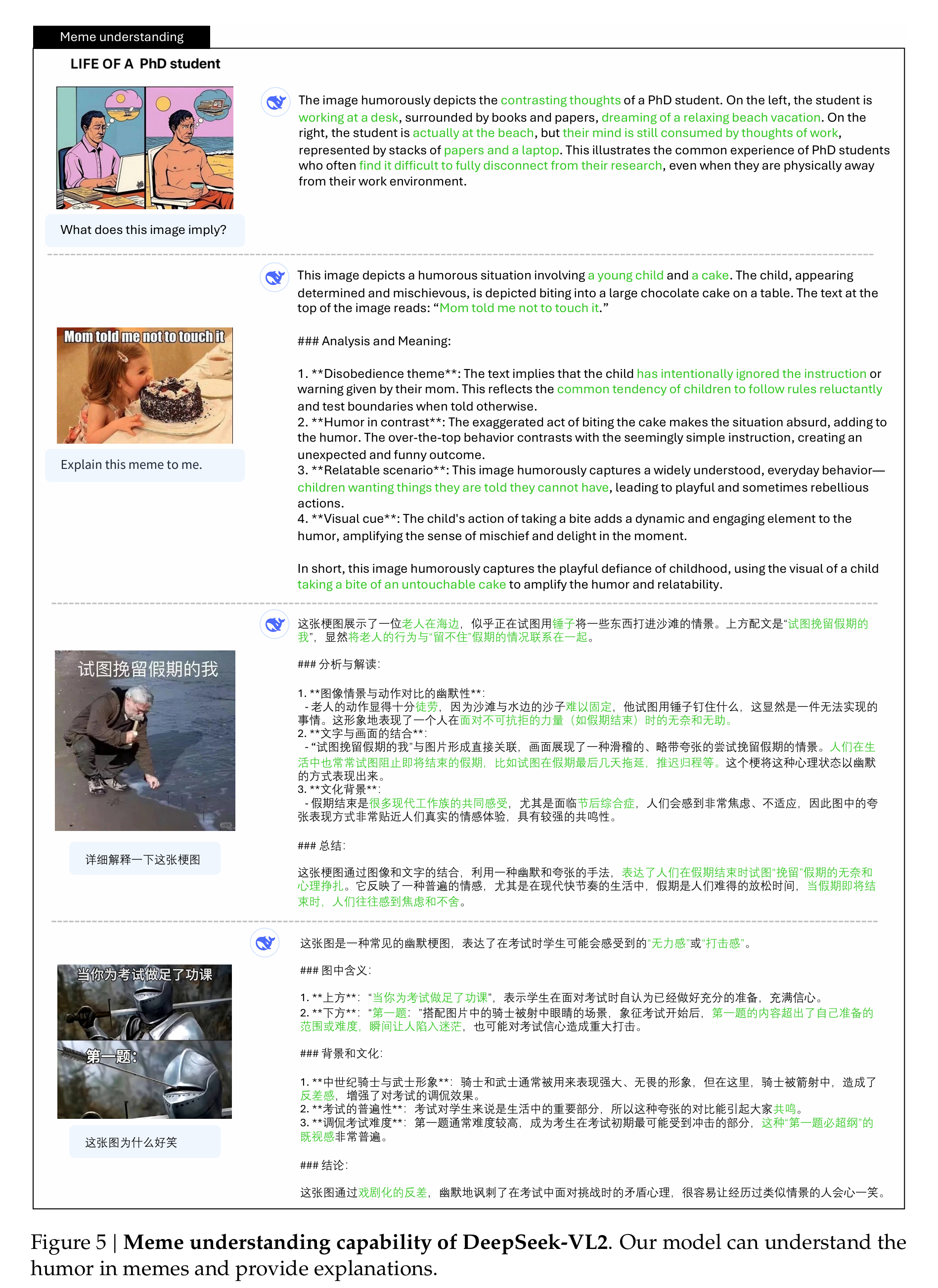
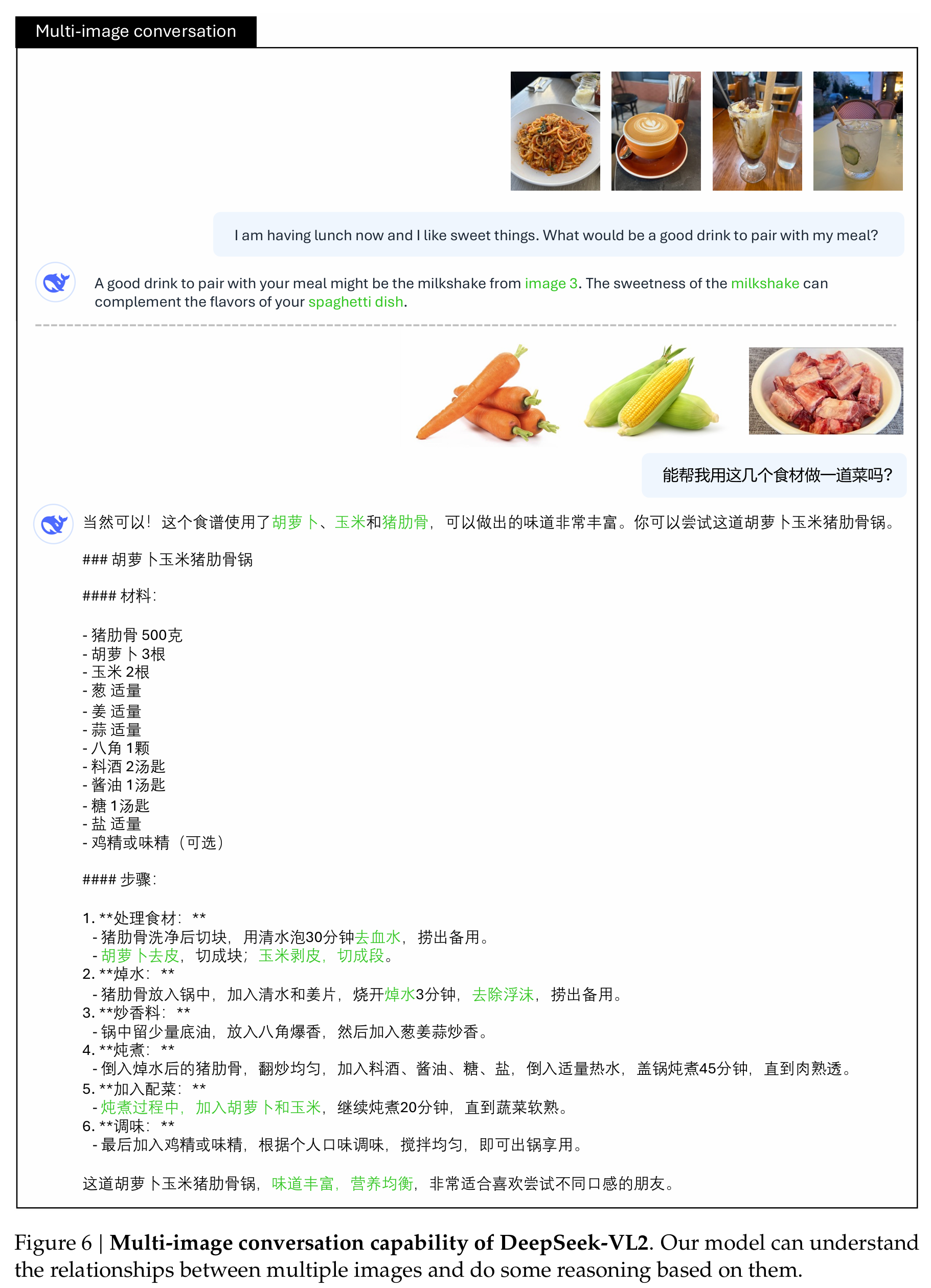
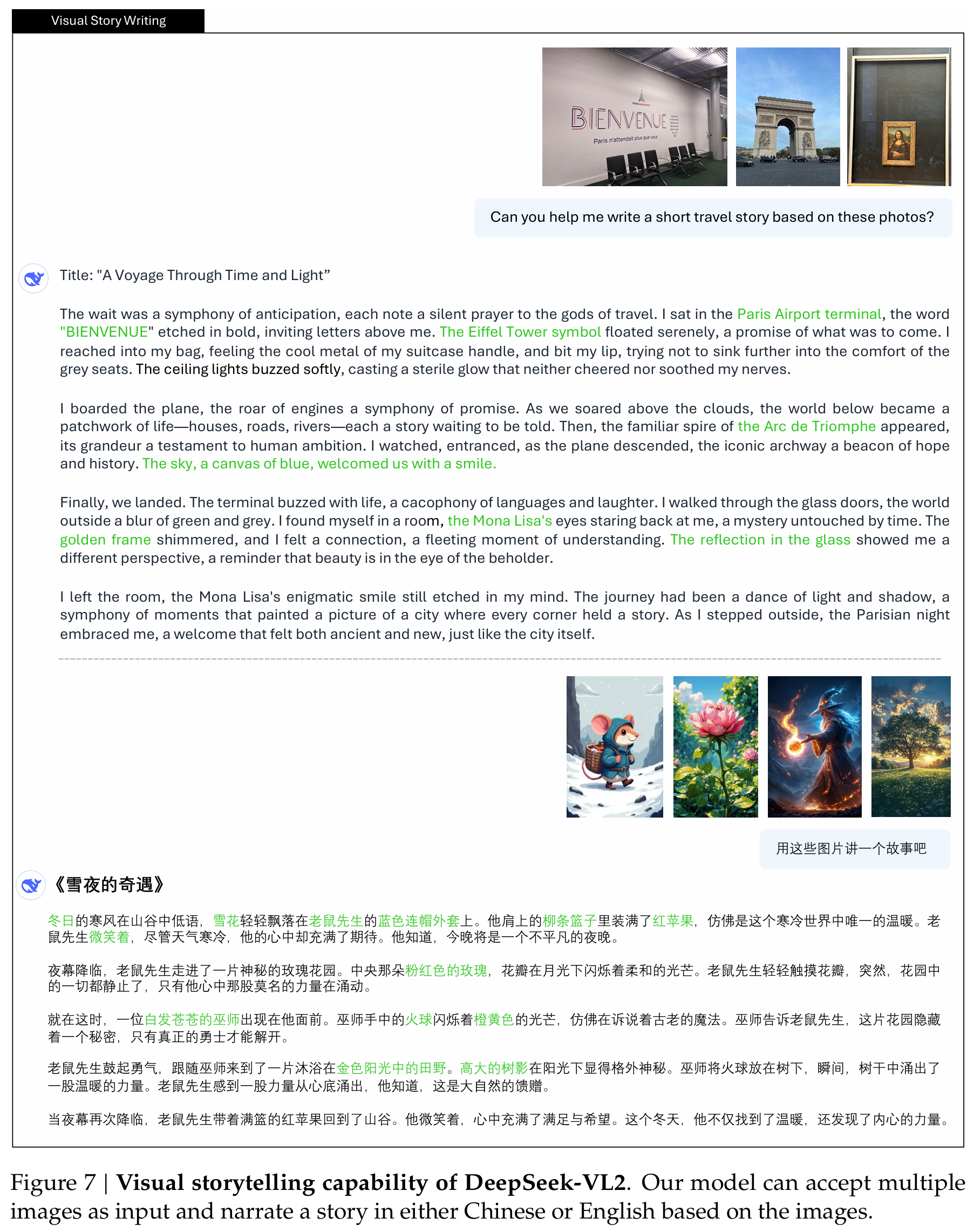
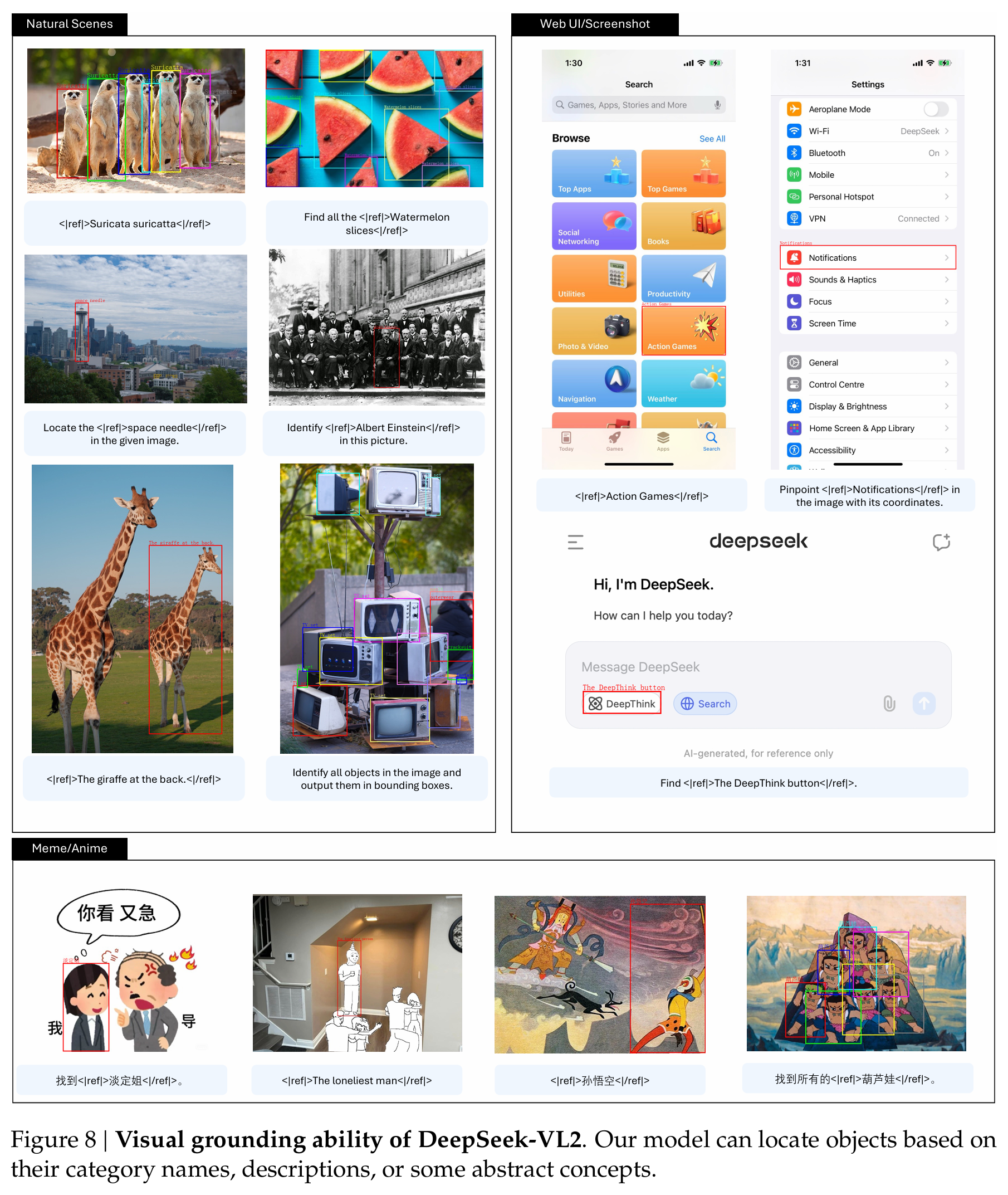
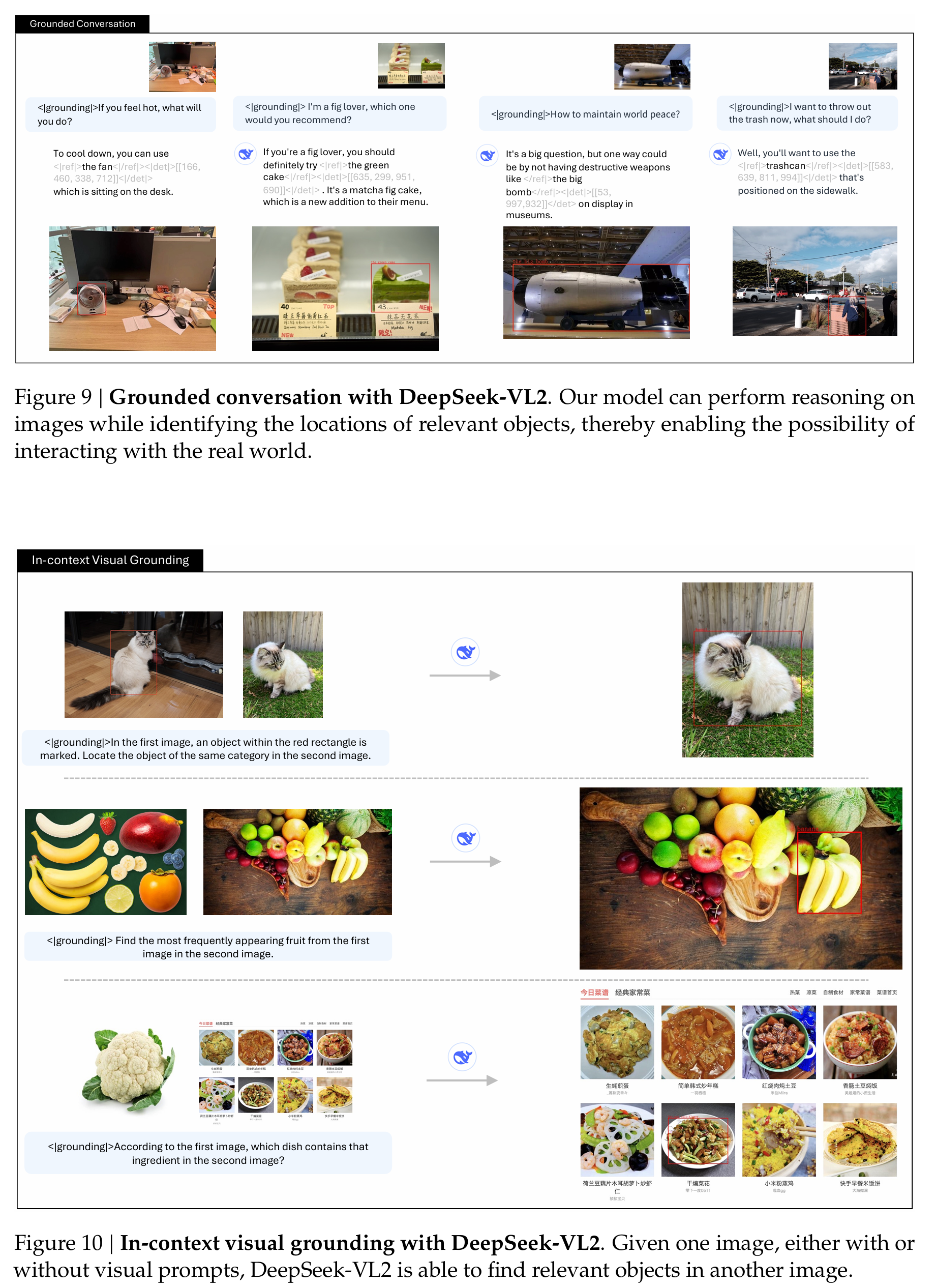
Conclusion
DeepSeek-VL2 represents a significant leap forward in vision-language modeling, achieving superior performance with fewer activated parameters. Its innovations in architecture, data construction, and training methodology position it as a leading open-source model for multimodal understanding.
Looking ahead, future work on DeepSeek-VL2 will focus on extending the context window, improving robustness, and enhancing reasoning capabilities. While the model excels in many areas, there is room for improvement in handling edge cases and expanding its generalization capabilities.
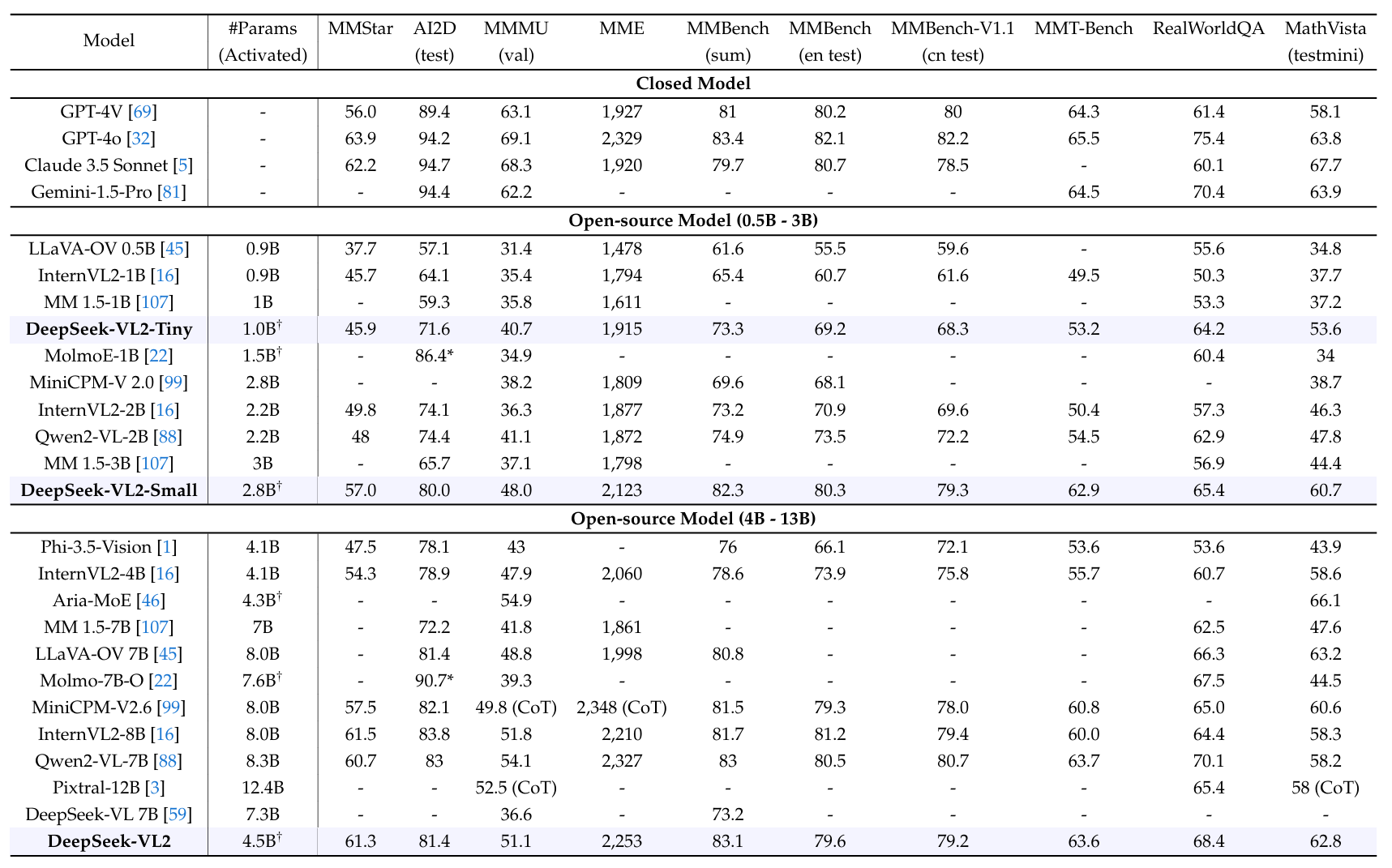
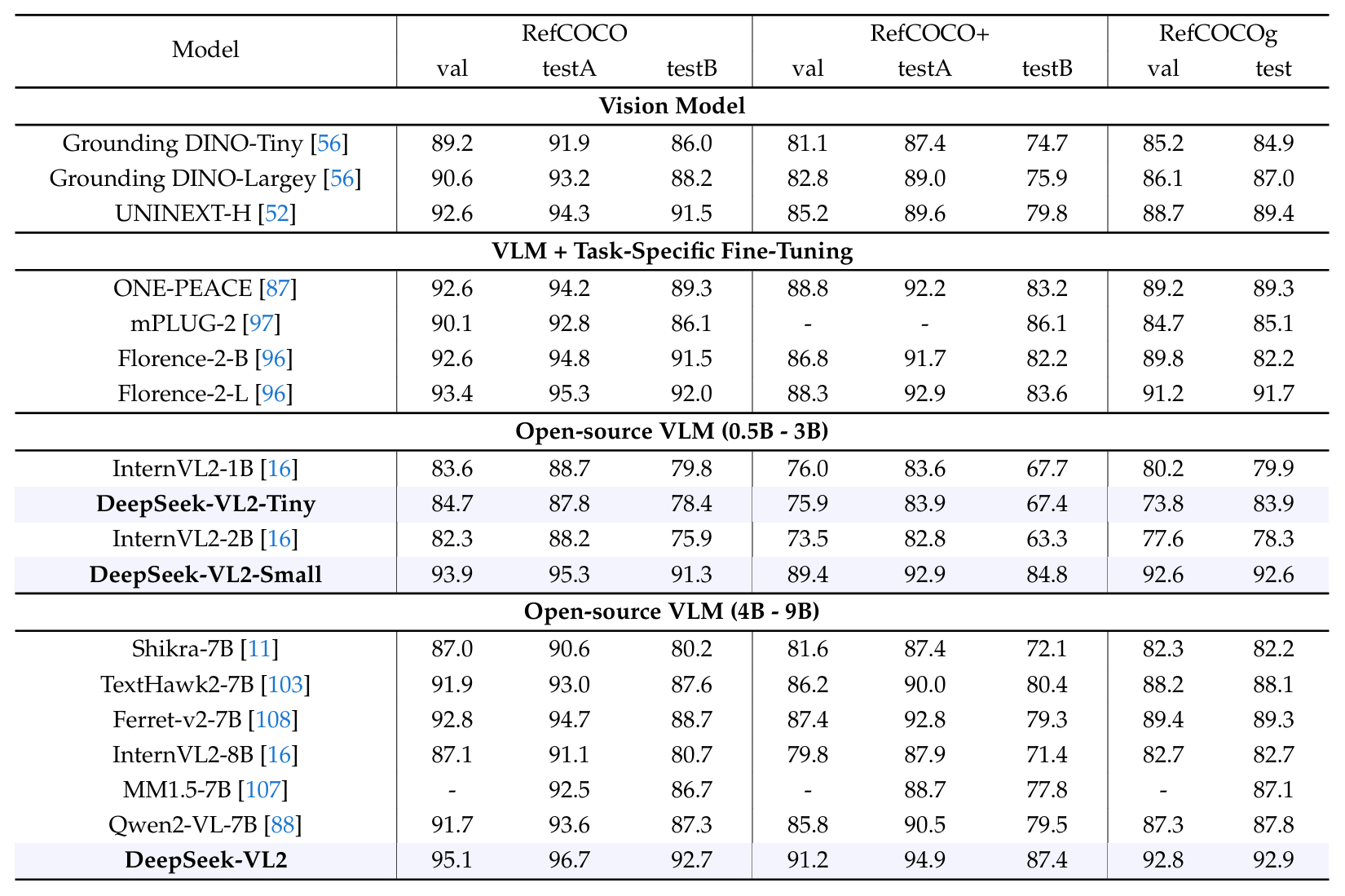
Key Takeaways
- DeepSeek-VL2 leverages a Mixture-of-Experts architecture to achieve state-of-the-art performance in vision-language tasks.
- Innovations like dynamic tiling and Multi-head Latent Attention enable efficient processing of high-resolution images and complex textual data.
- The model's training pipeline and curated datasets ensure robust multimodal capabilities, excelling in tasks like visual storytelling, meme understanding, and grounded conversations.
- Future advancements will focus on extending context windows and improving reasoning capabilities, solidifying DeepSeek-VL2's position as a leader in the field.
This article provides a comprehensive overview of DeepSeek-VL2, integrating relevant figures and tables to support the narrative. Let me know if you need further elaboration or adjustments!